HVAC management in office buildings: Rule-based strategies Vs. Artificial intelligence
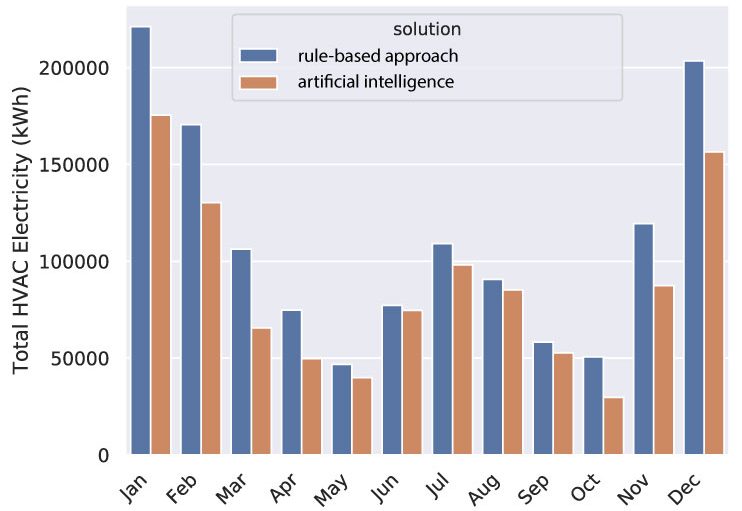
“Artificial intelligence” is everywhere in marketing materials and trade shows related to smart buildings. It’s true, Ai plays a key role in energy management, in HVAC management in particular as heating, cooling and ventilation are one of the largest energy consumers in most buildings, representing as much as 50-60% of the total energy consumption.
Better than just words, this article will show you the tangible benefits of our advanced machine learning algorithms on a building’s HVAC energy consumption.
First things first, we’ll benchmark our technology against a building model whose HVAC is managed by typical HVAC control rules. We call this way of managing the HVAC system via rules, “the baseline”.
The same building model will be used to compare:
1) Conventional HVAC control rules
Vs.
2) Artificial intelligence
The building we used to run our simulations on is known as a “medium office” as defined by the Department of Energy. The DOE identified its characteristics, with the purpose of having a building type that is representative of most commercial buildings across the US.
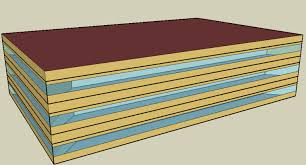
Here are the main characteristics of the building we optimized:
53,600 ft²
3 floors
33% window fraction
Building type: office
Energy types: gas and electricity
Desired outcome: thermal comfort at the best cost
Mechanical engineers design the HVAC system, with the goal of making it compliant with the building code (ASHRAE 55 and 62.1 in particular).
In a nutshell, ASHRAE-compliance means that the building’s indoor environment will need to provide comfort to its occupants (comfort being represented by PMV, see note below) and limit the number of predicted complaints.
Thermal comfort defined by ASHRAE 55
PMV (Predicted mean vote) is a metric designed to represent indoor thermal comfort and considers temperature, mean radiant temperature, humidity, airflow and even metabolic rate, and clothing insulation.
In this article, we chose to use a simplified approach where thermal comfort is simply defined by: “temperature stays between 71.5 and 75°F”
Another article will follow to see how our Ai performs with indoor conditions that stick strictly to ASHRAE requirements.
To learn more about PMV, check out here
After determining the HVAC system according to expected loads, comes the time to manage the HVAC equipment. In buildings equipped of a BMS (building management system), BMS contractors will implement the rules that manage the way HVAC equipment should react to variables, such as indoor temperature or outdoor conditions.
This is what we call the “conventional approach” or “rule-based approach” and represents our baseline.
Artificial intelligence becomes interesting if it outperforms the conventional approach by providing similar or better comfort with less energy.
Note: we assume here that the conventional approach manages to provide indoor thermal comfort, which is not always true, but this article focuses on energy consumption all other things being equal.
First, let’s check our Ai manages to provide thermal comfort
To get started, we asked Foobot Ai to maintain the temperature within a desirable range, eg between 71.5°F and 75°F maximum (or 22-24°C) while saving as much energy as it can.
We also added a constraint so that the temperature could not be changed by more than 0.5°C every 15 minutes.
As a result, the chart obtained below for a single day reveals the office space remains in a desirable temperature range. Furthermore, our Ai achieved a great thermal stability throughout the day:
Now, let’s look at the energy consumption achieved by the Foobot Ai compared to the baseline.
Get first-class indoor conditions in your office.
Learn more how our Ai improves thermal comfort while saving energy here
The same thermal comfort constraint, with minimum and maximum temperature thresholds as above, was required every day during a year, using the conventional approach (rule-based) on one hand, and our Ai on the other hand.
The charts below show the electricity and gas consumption used by cooling using electricity, heating using electricity and heating using gas:
The overall year round electricity consumption for heating and cooling shows that the Ai reduces energy consumption by 18.7%.
Here the constraint was set by a desirable temperature range. When we change the constraint to a desirable thermal comfort zone (set by PMV in ASHRAE 55), we manage to get as much as 38% in energy reduction. But let’s keep that for another article.
Type of HVAC control | Total energy consumption (GJ) |
Rule-based | 1439.60 |
Artificial intelligence | 1169.48 |
The Foobot Ai significantly outperformed the conventional approach for both gas and electricity consumption. It’s important to note that these results were obtained from the very same building model.
Also, as it is a building model, it runs perfectly.
In a similar real-life building, the Ai is expected to perform even better against a rule-based HVAC system, because it adapts itself. Rules can be very sophisticated, but they don’t evolve over time, as they are set in stone on day one.
For this reason, and because the Ai has gained so much experience in millions of varied situations during the time we trained it, rule-based buildings can’t save as much energy.
Applying Ai to complex topics such as energy management in buildings represent a tremendous opportunity to reduce carbon footprint and to save on costs. Your turn now: how do you optimize energy and thermal comfort in your buildings? Let us know in the comments below.
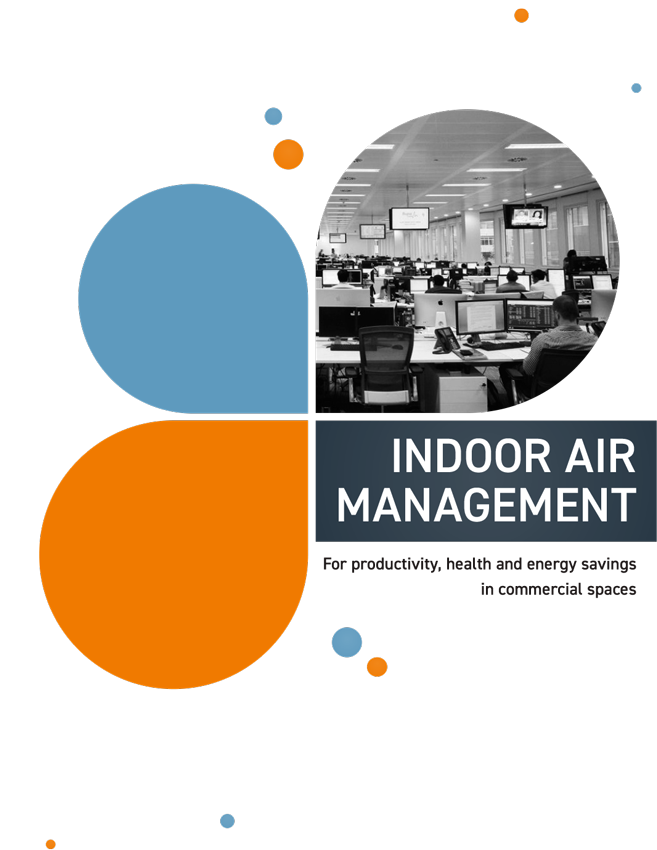
Indoor comfort and energy savings on autopilot
Thermal comfort and indoor air quality are very correlated to staff health and cognitive performance, studies say.
Since human resources are businesses’ largest expense, it’s time to give them the best.